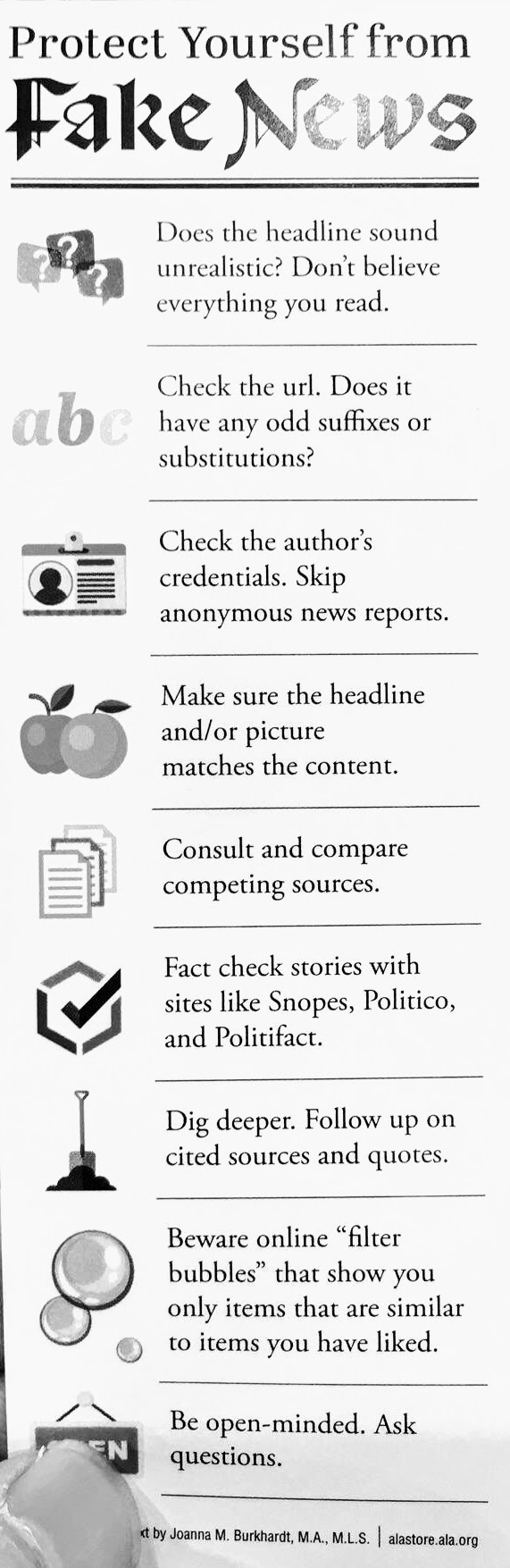
Back in school we learned that “Garbage in” leads to “Garbage out” – also referred to as GIGO. Today we see GIGO all over social media. We’ve been watching the erosion of information quality and its effects on society — the use of misinformation (fake news) and impersonations (fake accounts and bots) versus facts to form opinions and making decisions. This is compounded by the fact that it so easy to share information with so many people, in a very short amount of time. A recipe for disaster. I feel this problem is one of society’s biggest issues today. Some recent examples:
- Russian troll farm, 13 suspects indicted in 2016 election interference (The Washington Post)
- AI manipulation is on the rise (Richard Macmanus/Stuff)
- Why social media companies won’t kill off bots (Mashable)
And there are so many other examples…
See related Track Sessions at SXSW 2018: “Solving Fake News, Changing the Media, and the Future of Journalism: News & Journalism Track Sessions for SXSW 2018.”
Facebook and Twitter became the mechanisms and sources for garbage info in, and as a result the garbage that was and is being spread out to millions of people. These platforms allow for easy creation, sharing and monetization of information (and their audiences), but also for automation via the use of bots; see Twitter bots (Wikipedia).
Facebook and Twitter are information platforms — vast ecosystems that are like oceans that people navigate alone or with whomever they decide to navigate with. Who should bear the responsibility of who and what flows through these ocean-platforms? The answer is that it’s a shared responsibility between the social media platform providers, but primarily it is the responsibility of the people, consumers of these platforms. Yes, these platforms providers need to protect its consumers (ideally keeping the governments out of the equation), but consumers cannot be naive about what they consume. It is about common sense.
At the core of all this debacle is Trust. In social media, users define their circles of trust. These circles have inherit trust levels associated with them, for example:
Bob (human or bot) tells Alice about something. If Alice trusts Bob, she will accept the information provided, and form her own opinions. Alice then forwards to Jane. The cycle repeats. It is a recursive problem of trust and re-sharing information.
Let’s now look at an example of impersonation:
…if I follow Warren Buffet in Twitter, it is pretty safe to trust his expertise on what he says about investing for example, his 15 largest stock holdings. The issue is should I follow @WarrenBuffett or @itswarrenbuffet? The answer is that you want to follow his official verified @WarrenBuffett account. But many people don’t know what a Twitter verified account is and won’t research the account, and will trust (at least initially) their circle of friends, and will follow Mr. Buffet’s non-verified account if coming from such trusted friends.
People accept/believes a given source of information because either is (1) a source they know and trust, or 2) it is aligned with their believes or serves their purpose. The latter is hard to address — “closed minds” is hard to fix, but ignorance can be addressed.
So what can we Technologists do to help solve this problem?
Dive deep and apply the latest in tech and automated intelligence.
Let me begin by saying that I believe this issue can be addressed via continuous education which provides awareness. This should be supported by introducing automation and machine learning.
Is the concept of reputation a good way to address the issue of who to trust? Reputation leads to credibility which leads to trust.
— See Source (Springer).
Imagine a reputation system that indexes trusted sources, that tells you their background and biases, that would learn, identify and differentiate good (sources of) information from bots and impersonators, and from misinformation. A system that balances human and machine input, based on examples of what trusted sources are, biases and what misinformation is.
If providing such a system is successful, the Bob and Alice scenario should play differently:
Bob (human or bot) tells Alice about something. Bob is no longer trusted by Alice. Alice ignores the information from Bob, and won’t share it. The cycle repeats.
Why not give Alice the tools to (1) understand if Bob is a trusted source, and (2) to flag Bob as not trusted (if that is the case). In parallel, identify and eliminate bots and impersonation accounts. It is important that we start simple (KISS principle): educate consumers, start static, move on to automation and machine learning (this is a time-sensitive issue).
Twitter and Facebook have begun addressing this issue.
Facebook started something that resembles a Reputation system — the “i” or information button. But it seems to be a closed system. Also, have you noticed this button or even clicked on it? To tell you the truth, I didn’t notice this button until I did the research for this blog/essay. Perhaps they should go further:
- Make it more explicit. An example is color-coding such as red, yellow, and green based on the level trust.
- Allow people to flag content and sources of content. This can be risky as people are biased. Bias and mob fears can and will tilt the balance.
- Work with industry-supported groups that help determine what sources are trustworthy. Combine this with people’s input for additional check and balances.
- Extend this to “friends” — allow people to mark the trustworthiness of people in their own circles, with feedback back to the person being flagged.
- Perhaps create a consolidated reputation system that uses or is extended to all social media: Facebook, Twitter and so on.
- Apply machine learning to identify patterns and automate adjustments as needed.
- Constant awareness and education across the Facebook ecosystem.
Twitter is (finally) cracking down on bots (TechCrunch). As a side note I find interesting how people began complaining that their number of followers was drastically reduced — one person in my Twitter feed was complaining that it had lost more than 20K followers, which tells you how bad the bot situation is.
Browser plug-ins can also help with this issue of misinformation. One example is Fake News Detector. But the issue with browser plug-ins is distribution — the action required by the user to find and download the plug-in itself.
In conclusion, this is a hard problem to solve because of the human factor and bias. Bias by humans and mob fears. Bias by AI systems. The immediate answer is education, awareness and common sense. Key to solving this issue is cooperation between the social media platform providers and industry leaders providing independence, transparency and monitoring. Because of the vast amount of data, automation and supervised machine intelligence should be applied — a careful balance between human and machines. And remember, consume and support True Journalism.
-Carlos Enrique
Related:
- The Future of Truth and Misinformation Online (Pew Research Center)
- Fake News Detector browser plug-in
- ‘Fake News’ Reinforces Trust in Mainstream News Brands: Hits Reputation of Social Media Sources (Business Wire)
- “Solving Fake News, Changing the Media, and the Future of Journalism: News & Journalism Track Sessions for SXSW 2018.”
(Also published on Medium/@eortiz)